Objective:
Optimizing business profitability by maximizing customer retention and minimizing customer churn.
Introduction to Customer Churn Analysis in Machine Learning:
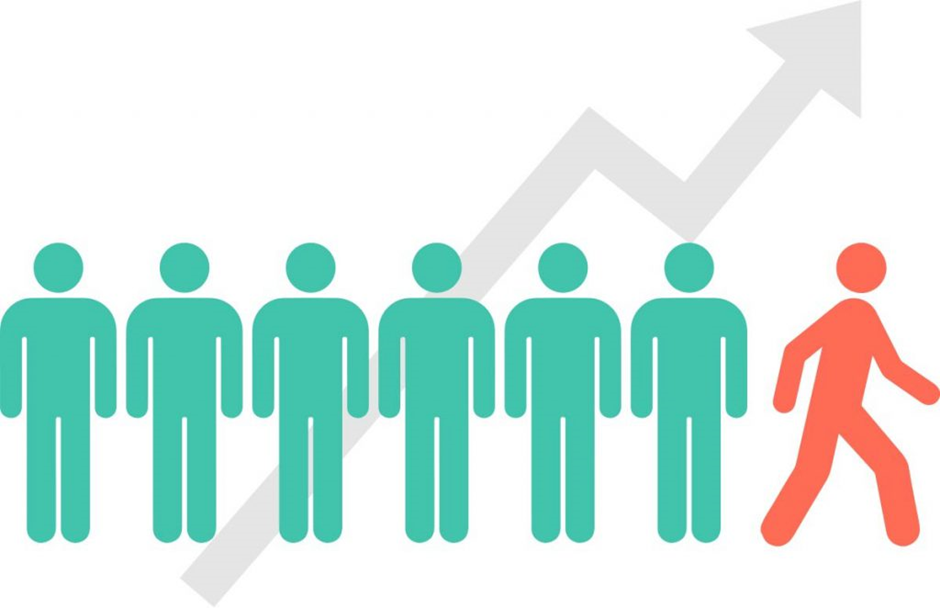
Customer churn, the phenomenon of customers stopping your service, is a major concern for many businesses. It costs more to acquire new customers than retain existing ones, making churn prediction a crucial area for investment.
Process:

1.Data Collection
- Data Sources:
- Customer demographics: Age, location, income, etc.
- Purchase history: Transaction details, frequency, amount spent.
- Support tickets: Number of tickets, nature of issues raised.
- Website interactions: Page views, product searches, time spent on site.
2.Data Preprocessing
- Challenges:
- Missing values: Customers might not provide all information.
- Data inconsistencies: Formatting errors, typos.
- Techniques:
- Imputation methods: Fill missing values with estimated values
- Outlier removal strategies: Identify and address extreme data points that skew analysis.
3.Exploratory Data Analysis (EDA)
- Highest churn rate segments: Identify groups with the highest proportion of customers leaving (e.g., by demographics, product categories).
- Most correlated factors: Find factors that have the strongest association with churn (e.g., purchase frequency, average order value, engagement metrics).
- Visualizations:
- Charts: Bar charts to compare churn rates across segments.
- Graphs: Scatter plots to visualize correlations between factors and churn.
4.Model Building and Evaluation
- Model Selection:
- Logistic Regression: Provides interpretable results, allowing you to understand why a customer is predicted to churn.
- Random Forests: Powerful ensemble method for accurate churn prediction.
- Evaluation Metrics:
- Accuracy: Overall model correctness in predicting churn.
- Precision: Ratio of true positives (correctly predicted churners) to total churn predictions.
- Recall: Ratio of true positives (correctly predicted churners) to all actual churners.
- AUC-ROC: Measures the model’s ability to differentiate between churners and non-churners (higher is better).
Applications:
Customer churn analysis has extensive applications across diverse industries, helping businesses understand why customers leave and develop strategies to retain them. Here are some real-world examples:
1.Telecom:
- Identify subscribers likely to switch providers: Analyze call patterns, data usage, and service plan changes to identify customers who might be looking for better deals elsewhere.
- Target churn reduction campaigns: Offer targeted promotions or loyalty programs to high-risk subscribers based on their usage patterns.
2. Retail:
- Predict customer abandonment during online checkout: Analyze incomplete purchases and identify factors causing cart abandonment.
- Personalize product recommendations: Recommend products based on past purchases and browsing behavior to increase customer engagement and reduce churn.
3. Media & Entertainment:
- Analyze user engagement with streaming services: Identify patterns in content consumption to predict churn.
- Target churn reduction campaigns: Offer personalized recommendations, exclusive content, or free trials to re-engage users at risk of cancelling subscriptions.
4. Financial Services:
- Identify customers at risk of account closure: Analyze account activity and identify customers with low balances, infrequent transactions, or missed payments.
- Proactive outreach with personalized financial advice: Offer relevant financial products or services to address customer needs and prevent churn.
5. Healthcare:
- Identify patients at risk of dropping out of treatment plans: Analyze appointment adherence, medication refills, and communication patterns.
- Targeted outreach with personalized health reminders or support programs: Encourage patients to stay on track with their treatment plans and improve health outcomes.
Advantages of Customer Churn Analysis:
Customer churn analysis offers a wealth of advantages for businesses, helping them retain valuable customers and boost their bottom line. Here are some key benefits:
1. Identify root causes of churn:
Instead of just knowing customers are leaving, you can pinpoint the specific reasons behind their departure. This might be product shortcomings, poor customer service, pricing issues, or competitor influence.
2. Prioritize retention efforts:
By understanding which customers are at risk of churning, you can focus your resources on the most valuable ones. This allows for targeted interventions and personalized engagement strategies.
3. Improve customer lifetime value (CLTV):
Retaining existing customers is significantly cheaper than acquiring new ones. Churn analysis helps you maximize the value each customer brings by keeping them satisfied and engaged over time.
4. Enhance customer experience:
Analysing churn reasons can reveal areas where your customer experience falls short. This allows you to identify and address pain points, leading to happier and more loyal customers.
5. Predict future churn:
Advanced analytics can forecast which customers are likely to churn, allowing you to proactively reach out and offer solutions before they leave. This can significantly reduce churn rates and save revenue.
6. Optimize product and service offerings:
Churn insights can help you identify features or functionalities that customers find lacking or unnecessary. This helps you prioritize product development and improve your offerings to better meet customer needs.
7. Inform pricing strategies:
Analysing churn reasons can reveal price sensitivity or dissatisfaction with specific pricing structures. This helps you adjust your pricing model to retain customers and optimize revenue streams.
8. Benchmark against competitors:
By comparing your churn rate and reasons to industry benchmarks or competitor data, you can identify areas where you need to improve and learn from best practices.
9. Strengthen customer relationships:
Addressing churn reasons demonstrates your commitment to listening to customers and taking action to improve. This fosters trust and strengthens relationships, leading to increased loyalty and advocacy.
10. Improve overall business performance:
Reduced churn directly translates to higher revenue, increased profitability, and improved financial health. This can fuel further growth and investment in customer-centric initiatives.
Conclusion:

Developing targeted interventions based on predicted churn risk and churn reasons, personalized interventions can be designed. This could involve targeted discounts, exclusive offers, improved customer support, or personalized product recommendations.